
- This event has passed.
Ph.D. (Engg): Behaviour Modelling of Non-Cooperative Space Objects and Strategies for Decision Support in Space Situational Awareness
April 16 @ 3:00 PM - 5:00 PM
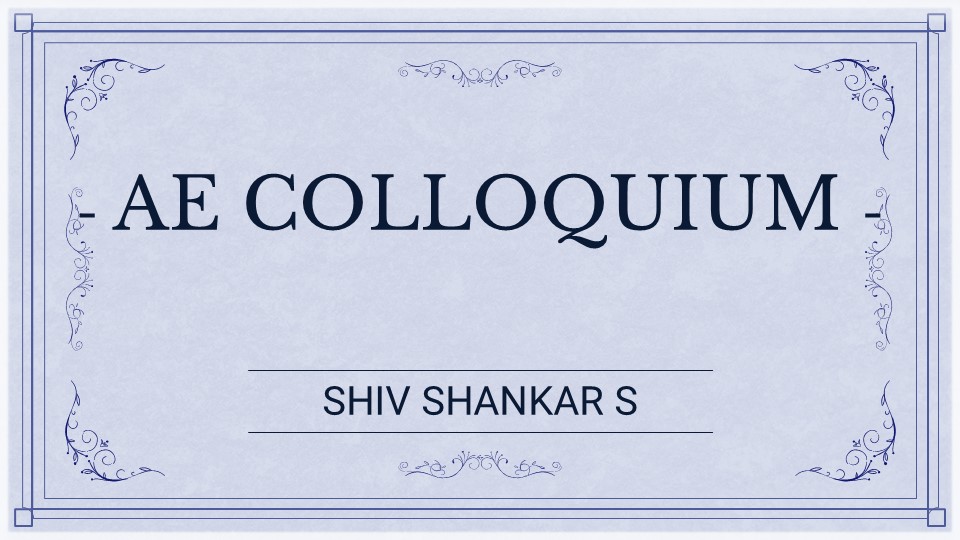
In this modern era, Space is vital for a Nation’s prosperity and without space, many critical functions would simply stop working. The increasing number of satellite launches in recent times, is congesting the space environment. Space is also becoming an increasingly contested environment from the perspective of non-civilian applications of satellites. The civilian and non-civilian space applications mandatorily require a complete awareness of the space environment before taking any operational decisions. Space Situational Awareness [SSA] is the comprehensive knowledge of Resident Space Objects [RSOs] which may include satellites, rocket bodies, debris, and the ability to track and understand their behaviour. Space objects can be majorly categorized into two broad types, cooperative space objects and non-cooperative space objects. A noncooperative space object is defined as a non-friendly object in space and can be perceived as a threat if it performs anomalous maneuvers in space. Modelling pattern-of-life of non-cooperative space objects is an essential requirement of SSA. Maneuvers of non-cooperative satellites is an important event of interest in their life pattern. In this thesis, we investigate the behaviour of various classes of satellites through data driven modelling. We also study the threat perception from non-cooperative space objects to space assets of our interest. There are four key areas, in which the thesis has significantly contributed. The first area deals with investigating, exploring and modelling pattern-of-life of non-cooperative space objects. We have crafted data-driven solution methodologies from time series analysis, machine learning, deep learning to suit specific requirements. The second area pertains to the maneuvers of non-cooperative space objects. Identifying them, helps in analyzing their behaviour. Since there may be numerous non-cooperative space objects and not all maneuvers of non-cooperative space objects may be threatening in nature, it is essential to segregate routine maneuvers needed by a satellite to maintain its orbit from anomalous and abnormal maneuvers which may be perceived as threat. In this thesis, we designed an approach to segregate benign and regular pattern-of-life maneuvers of non-cooperative space objects from their orbital data . The routine pattern-of-life maneuvers of satellites are events of interest, but are infrequent and hence the non-maneuver class was observed to be far more numerous than the maneuver class label in the dataset. Through this thesis work, we have applied Synthetic Minority Oversampling Techniques (SMOTE) and its variants to handle the imbalance in dataset available for classification. Different missions of cooperative civilian satellites in Low Earth Orbit (LEO) space regime were evaluated to prove the efficacy of the approach. The third area of contribution is in developing methodologies to estimate the threat perception for Geostationary Orbit (GEO) space regime. Modelling pattern-of-life of non-cooperative GEO satellites helps to identify anomalous behaviour and is essential for SSA. Additionally, given a satellite of interest, an assessment of the area of influence of neighbourhood satellite operations is critical for assessment of threat. Nearest neighbour search is a fundamental problem in computational geometry and we studied two major concepts of computational geometry , the Voronoi diagram and the Delaunay triangulation in detail and crafted algorithms to assess threat in the GEO space regime. The last area of contribution is with scheduling the limited and costly ground based sensors to monitor the large number of space objects. There exists a problem of gaps in the available orbital data of noncooperative satellites. Moreover, the satellite maneuver (event of interest) occurrence information of some samples may be lost, due to noise in the ground sensor observations or due to observation window limits or losing tracks. Conventional machine learning regression methods are not suited to be able to include both the event and time aspects as the outcome. The conventional models are also are not equipped to handle censored examples (incomplete data due to non-observability). Therefore, in this thesis, we devised a solution methodology by applying Time-to-Event data analysis (survival analysis) techniques to assess whether a satellite maneuvered, that is whether the event of interest occurred or not, and also estimate when the next maneuver would occur. We have explored a variety of approaches including Cox proportional hazards model, Weibull distribution model, Kaplan-Meier model, Nelson-Aalen model, Random survival forest, Survival Support Vector Machines, Gradient boosted survival analysis and Deep learning based survival analysis. Detailed experimental results based on real life satellite orbital datasets are presented to bring out the effectiveness of the solution methodology. To summarize, the thesis contributes by developing a space situational awareness system to achieve behavioural modelling, classification and characterization of space objects of interest, maneuver classification, anomaly detection and threat assessment through data driven methodologies.
Speaker: Shiv Shankar S
Research Supervisor: Debasish Ghose